Enhancing Ethical Profanity Analysis in Text Mining
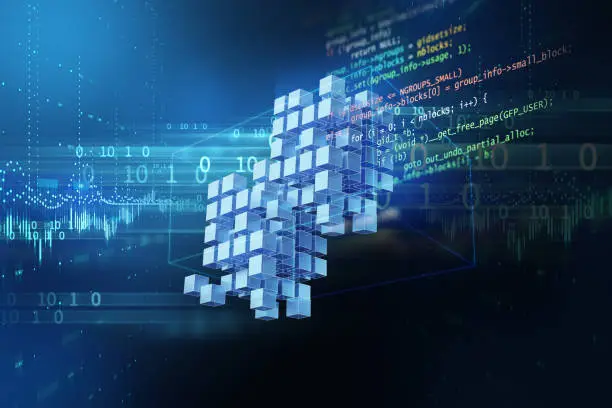
Profanity analysis in text mining is like having a filter for bad words in a giant library of online text. This can be used to maintain a certain level of decorum in online spaces by identifying and potentially filtering out offensive language.
While this can contribute to a more respectful environment, it's important to acknowledge that profanity can also hold emotional weight. Analyzing its use can offer insights into user sentiment, helping us understand if people are expressing satisfaction, frustration, or something else entirely. Ultimately, profanity analysis is a tool with potential benefits and drawbacks to consider.
Let’s understand the ethical challenges of profanity analysis and how to address them for a better online environment for all.
Ethical Challenges in Profanity Analysis
Addressing the challenges of profanity analysis in text mining involves transparent practices, diverse training data, and constant scrutiny of algorithms. Therefore, it is important to prioritize user privacy, mitigate biases, and minimize the risk of false positives to ensure fair and respectful profanity analysis.
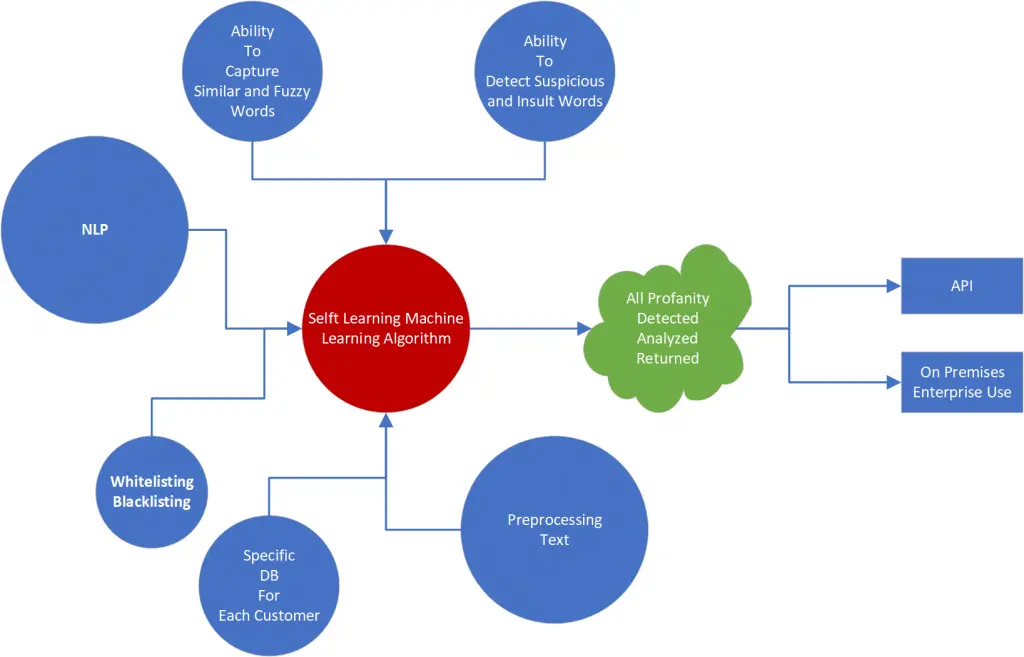
1. Privacy Concerns
Collecting and storing user data that contains inappropriate language raises privacy worries. Imagine if private conversations were monitored without consent.
A messaging app may be analyzing chats for bad language without users knowing. It violates privacy rights and makes people uneasy about their data being monitored without permission.
2. Bias and Fairness Issues
Profanity detection algorithms can be biased as they might be unfairly targeting certain groups or cultures. Words may have different meanings or acceptability in different languages.
For instance, a word considered offensive in one culture or language might be harmless in another. Profanity detectors may not consider this before flagging and unfairly taking action on users who use such words.
For example, users on an online chess forum realized their private chats on the platform were being monitored and unfairly flagged for profanity. Several phrases in different languages were translated and flagged as offensive.
3. Potential Harm from Misclassification
Inappropriate words can often lead to censorship or unfair consequences. Innocent words might be flagged and cause unnecessary trouble. For example, if a comment is mistakenly flagged as offensive, the platform might delete it or the user may face punishment. It could restrict free expression and can damage reputations.
Transparency and Accountability
Transparency is about sharing accurate information with the stakeholders. Accountability, on the other side, complements transparency by establishing guidelines to ensure the responsible use of the analysis tools and algorithms.
1. Transparency in Algorithm Development
Online platforms must be transparent while using profanity filters. Users should know how profanity detection algorithms are created, what data they use, and how they make decisions. For instance, a company might explain the process of selecting which words are considered profane and why.
2. Accountability Measures
Think of it as having someone watching to make sure everyone plays fair. Regular audits are important to ensure that profanity analysis tools are used responsibly. For example, an independent organization might review a platform's practices to ensure they comply with ethical standards and legal requirements.
Data Privacy and Security
Companies can prevent unauthorized access to user information by having strong security practices in place. Some of these measures include encryption, access controls, and compliance with data protection regulations like GDPR and CCPA.

1. Encryption and Secure Handling
Companies can use encryption techniques to protect user data and to maintain its integrity. Encryption protects data if someone tries to access it without permission. Additionally, strict access controls ensure that only authorized users can view sensitive information.
2. Compliance with Regulations
Regulations like GDPR and CCPA are meant to protect user rights and privacy. Companies need to follow specific rules to make sure they handle data responsibly. For instance, companies must obtain user consent before collecting or using their data and provide options for users to access or delete their data upon request.
Mitigating Bias and Discrimination
Using vast datasets for training, such as samples from different cultures, can help algorithms understand a wider range of words and their meanings. This can help reduce biases while enhancing the accuracy and fairness of profanity detection systems.
1. Diverse Training Data
Using diverse training data means including words and phrases from various cultures and backgrounds. This helps the profanity detection model understand different contexts and meanings. For example, if a word is considered offensive in one community but not in another, the model can learn to distinguish between them.
2. Fairness-Aware Algorithms
The algorithm ensures that the profanity detection model treats all words and users fairly, regardless of their background. For instance, these algorithms can be designed to give equal weight to words from different demographics, preventing bias against certain groups.
3. Addressing Discrimination
Profanity analysis should not unfairly target a specific community. For example, words associated with a particular ethnicity, gender, or sexual orientation should not be automatically marked as profanity without proper context. Addressing discrimination ensures that profanity detection is fair and respectful to all users.
User Consent and Control
By obtaining informed consent and providing users with control over their data, platforms can build trust and respect users' privacy rights. It's all about giving users the power to make decisions about their own data and ensuring transparency in how it's used.
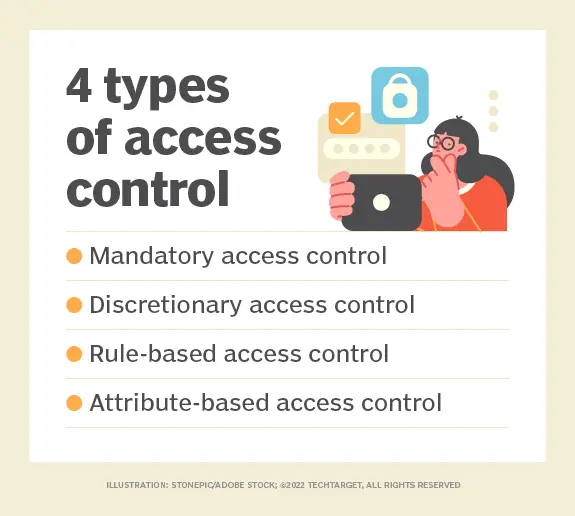
1. Informed Consent
When it comes to profanity analysis in text mining, it's important for users to know how their data will be used. For example, before signing up for a social media platform, users could be informed about the platform's profanity analysis practices and asked if they consent to it. This allows users to make an informed decision about whether they want to use the platform or not.
2. User Control
Imagine having a remote control for your data. Users should have options to control their data and decide if they want to participate in profanity analysis. Platforms could provide settings where users can choose to opt out of profanity analysis if they prefer. This gives users the freedom to decide what happens with their data.
Imagine if you could adjust privacy settings for profanity analysis, like a remote for your data. Platforms could offer opt-out options, giving you the power to decide if your data is analyzed for swear words. This enables users to define their own comfort level and privacy preferences.
Continuous Monitoring and Adaptation
Continuous monitoring and adaptation are essential for ensuring that profanity analysis remains effective and up-to-date. By staying proactive and responsive to changes in language usage and user feedback, we can maintain the quality and reliability of profanity detection systems.
1. Regular Updates
Updating profanity analysis models frequently is important because language changes over time and new words or meanings may emerge. For example, slang terms that were not considered offensive in the past may become so in the future. By updating the models regularly, we can ensure they stay accurate and effective in identifying profanity.
2. Feedback Mechanisms
Feedback allows users to share their thoughts on the performance of profanity analysis tools. By allowing users to report if a word was incorrectly flagged as profanity, it is possible to improve the accuracy of the model. Listening to user feedback can help identify areas for improvement in the analysis algorithm.
Conclusion
In summary, the ethical complexities of profanity analysis in text mining involve balancing privacy, fairness, and accuracy. Ensuring transparency, accountability, and user control are essential to overcome these challenges.
For a better future, it is important to refine algorithms through diverse training data and continuous monitoring while also prioritizing user consent and feedback. For those interested in advancing AI solutions, MarkovML offers cutting-edge tools and expertise. Companies can build a safer and more welcoming digital space using these tools.
Let’s Talk About What MarkovML
Can Do for Your Business
Boost your Data to AI journey with MarkovML today!