The Power of Self-Service Data Analytics for Smarter Decisions
Imagine a world where key corporate decisions are made seamlessly, driven by self-service Data Analytics at the helm —sounds promising, right?
In the realm of transformative advancements, global healthcare technology giant Medtronic exemplifies a shift towards self-empowerment in data analytics. From initially addressing 100% of procurement users' analytics inquiries, Medtronic's data team now handles only 20% of these questions.
A staggering 80% is easily managed by the business users themselves, marking a paradigmatic embrace of self-service data analytics for more informed decision-making. This compelling transition underscores the burgeoning influence of self-sufficiency in harnessing the potential of data analytics for smarter decisions.
In this blog, we will look into what self-service data analytics is, and we will unfold its advantages, shortages, effective methods, practical examples, and forthcoming trends.
Understanding these aspects will equip knowledge workers like yourself with the capability of leveraging this cutting-edge technology, reinventing the way you frame strategies, devise plans, and enforce decisions. So, let's begin.
The Rise of Self-Service Data Analytics

The digital world is in the middle of a massive shift, with self-service data analytics leading the way. This innovative approach helps knowledge workers like you to examine data independently, helping you make quick and informed decision-making without the requirement of consulting a data scientist.
In doing this, self-service data analytics facilitates widespread data access, minimizes obstacles, and fosters an environment for growing business operations.
- Enhance your Efficiency: With the help of self-service data analytics, knowledge workers can effectively conduct their own data analysis rather than waiting for data scientists. This helps with the conduct of immediate analysis and speeds up the process of decision-making with correct insights.
- Empowering the workforce: Utilizing self-service analytics empowers organizations to boost their team's independence, sparking innovation and increasing efficiency.
- Utilization of Optimal Resources: Providing knowledge workers independence to manage basic data analytics tasks grants data researchers the bandwidth for more thorough assignments, leading to an efficient distribution of resources.
- Promoting Data-Centric Culture: The implementation of self-service analytics enables organizations to foster a culture focused on data, encouraging transparency, collaboration, and data-driven decision-making.
An assessment by Zion Market Research suggests that the global self-service analytics market is expected to climb to USD 62.39 billion by 2028, indicating a compound annual growth rate (CAGR) of 7.6% from 2021 to 2028. This demonstrates the growing acceptance and reliance on self-service analytics in various industry sectors.
Benefits of Self-Service Data Analytics

When it comes to self-service data analytics benefits, one crucial factor that frequently goes unnoticed is the role of the user. Hence, equipping knowledge workers with actionable data-derived insights has emerged as a frontrunner for forward-thinking establishments.
Let's have a look at some of the benefits that encompass the analysis of self-service data.
1. Empowering Non-Technical Users
With self-service data analytics, every team member can become a data explorer, even without extensive technical expertise. These tools democratize data, allowing knowledge workers to navigate the vast amount of data effortlessly.
The key to this transformation lies in the tool's accessibility, user-friendliness, and the possibility of customization combined with personalization. These features allow for tailored data interpretations that go beyond standard representations.
2. Faster Insights and Decision-making
With the increasing drive for data-driven practices, faster generation of insights and decision-making have become pivotal. Self-service data analytics tools, namely their robust algorithms and analytics models, turn vast amounts of raw data into meaningful, actionable insights swiftly.
Rapid insight generation subsequently allows for speedier decision-making, promoting agility within the organization.
3. Enhanced Collaboration
Collaboration is a crucial aspect where self-service data analytics truly shines. By enabling users to share analytics results conveniently, team collaboration gets a significant boost. It provides a common ground for all stakeholders to engage, facilitating dialogue driven by data-derived insights.
4. Reduced Dependency on IT
One of the conveniences of self-service data analytics is the reduced dependency on IT. End-users have greater control over their analytics journey, all while freeing up IT resources for more critical tasks. The ability to make adjustments or dig deeper into data without waiting for IT makes it a boon.
5. Improved Resource Allocation
By circumventing the traditional ways of maintaining data, businesses can improve resource allocation. Resources previously spent on data extraction and preparation can now be redirected to more profitable channels.
6. Agility in Decision-Making
Self-service data analytics tools bring agility to decision-making. A company’s ability to react swiftly and modify plans based on new information is enhanced by the up-to-minute insights delivered by self-service tools. It means quicker resolution of issues, smoother process changes, and higher potential for overall success.
Challenges with Self-Service Data Analytics
Navigating the world of self-service data analytics offers a lot of advantages. However, it's vital to acknowledge and address potential challenges along the way. This encompasses ensuring the highest quality of data, upholding stringent measures towards data privacy and security, filling in the gaps in user training and skill set, meeting governance and compliance requirements, and integrating smoothly with existing systems.
Let's have a look at some of the self-service data analytics challenges here:
1. Data Quality and Consistency
Maintaining the integrity and uniformity of data input is paramount. Evaluating data that are unreliable or inconsistent could result in skewed outcomes and potentially erroneous decision-making processes. For robust and reliable analytics, companies need to establish a data governance framework that can monitor and maintain data standards.
- Implement systematic checks to verify data accuracy.
- Take measures to guarantee that your data is both current and pertinent to your requirements.
- Set clear instructions to maintain uniformity across your data sets.
2. Data Security and Privacy
It's crucial to respect data privacy and ensure its security for effective data management. Ignoring this can lead to big fines and damage to the organization's reputation. To prevent these problems, it's essential to follow data security procedures strictly.
Steps like encoding data can improve security, and strict controls over who can access the data ensure it's only available to the right people.
- Ensure robust encryption for critical data and apply rigorous access controls.
- Adopt a data analysis method that places a high importance on protecting privacy.
3. User Training and Skill Gaps
To fully harness the power of self-service data analytics tools, users may require adequate training and resources for constant skill development. Solving this issue could mean conducting regular training programs and supplying materials that facilitate continual learning.
- Provide regular training sessions and skill-building workshops.
- Encourage self-learning through online resources and guides.
4. Governance and Compliance
Companies must establish rules or governance and consistently follow laws and standards, known as compliance. They need to stick to these compliance measures while keeping up with changes in laws and regulations.
- Implement internal controls and audits for data quality.
- Stay updated with regulatory changes and adapt accordingly.
5. Integration with Existing Systems
Integrating self-service data analytics with existing systems can be challenging. Seamless integration constitutes data flow from multiple sources, which requires technical planning and execution.
- Plan architectural designs that accommodate data analytics integration.
- Prevent potential disruptions with thorough testing.
Best Practices for Implementation
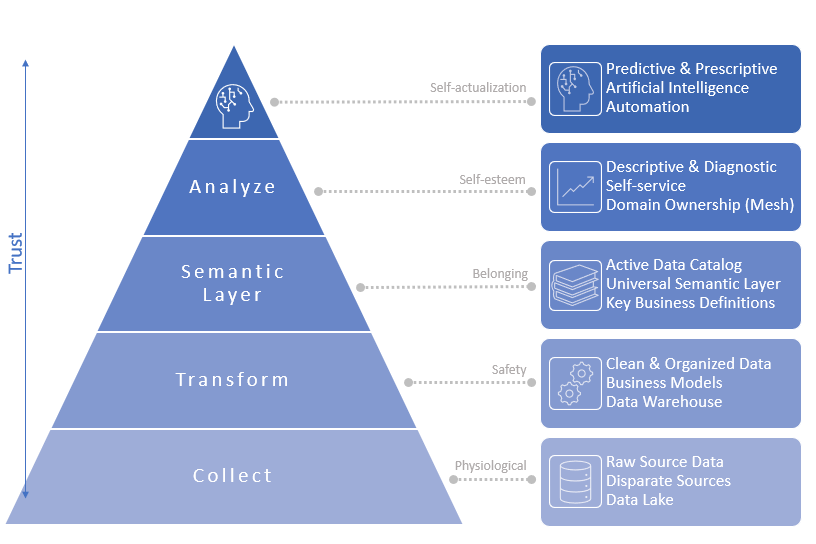
Embracing self-service data analytics is transformative, but implementing it effectively requires a strategic approach. Below are the self-service data analytics best practices to ensure successful implementation:
- Choose the correct Self-Service Data Analytics Tool: The effectiveness of a self-service analytics tool greatly hinges on its suitability to an organization's specific needs. It's important to opt for tools with user-friendly interfaces and features like foresight analytics, smart data exploration, and data illustration capabilities.
- Maintaining High Data Quality: The accuracy of decisions made with the help of self-service data analysis depends upon the quality of data used. Always run checks on your data to make sure it's correct. This helps to make better decisions. According to a 2021 report by Gartner, businesses lose an estimated $15 million in a year due to poor data quality.
- Upholding Robust Data Governance Policies: It's important to apply strict data governance rules to set up protective actions for sensitive data and comply with data privacy regulations. A comprehensive governance framework ensures data consistency and accuracy, in addition to enhancing data safety.
- Providing Continuous and Consistent Training: To maximize the benefits of self-service analytics tools, knowledge workers within the organization must be proficient in their usage. Hence, regular training sessions are paramount to boosting staff confidence, thereby promoting effective tool adoption.
- Fostering a Data-Driven Organizational Culture: Incorporating a data-driven decision-making culture enables employees to utilize self-service analytics in their roles progressively. According to a study by the Business Application Research Center (BARC), organizations that emphatically implement data-driven methodologies stand a chance to improve their customer acquisition rate by as much as 23 times.
By following these best practices, organizations can reap the full benefits of self-service data analytics effectively.
Real-World Success Stories
When we look at the self-service data analytics example, a case in the retail sector stands out. A perfect example of using self-service data analytics effectively is seen in Wayfair, a top online shopping company for home goods.
Wayfair has effectively leveraged self-service data analytics, democratizing the accessibility and application of data across various departments. As a result, analytical solutions are now developed and implemented more quickly, leading to faster insights and agile decision-making.
The benefits were notable —a significant decrease in lead times for data-driven initiatives and increased responsiveness to market changes.
Future Trends and Innovations
The web landscape is continually evolving, shaped by the latest self-service data analytics trends and innovations. As the reach of data democratization expands, advanced technologies continually open new possibilities. Let's have a look:
- Simplified Analytics: With modern tools such as data analysis, artificial intelligence, and machine learning, simplified analytics reinvents how we handle and understand data. This innovation speeds up decision-making by offering fast, valuable insights.
- Data Visualization: Understandably, showing data is very important in data analytics. New technologies like virtual reality and enhanced reality are now used to create eye-catching ways to view data.
- Integration of AI and ML: Using artificial intelligence and machine learning in self-service data analytics is growing quickly. This means users can effortlessly make and understand reports, even if they don't have experience with cloud services or coding.
Conclusion
The world of decision-making is changing with the introduction of self-service data analytics. Platforms like MarkovML offer powerful tools such as data management, AI application development, automated machine learning workflows, ethically responsible AI, and team collaboration features. These tools can speed up this process and produce better outcomes.
Empower your decision-making process like never before by exploring the dynamism of data with MarkovML, a state-of-the-art AI platform. Seamlessly navigate through the intricacies of data analytics, ensure absolute transparency, and significantly elevate your team's productivity.
Explore the untapped opportunities of your data with MarkovML today.
FAQs
1. What is Self-Service Data Analytics?
Self-service data Analytics constitutes a segment of business intelligence that equips end users or knowledge workers with the capability to execute analytical queries independently and create reports without needing direct aid from IT or data specialists.
2. What are some of the challenges of Self-Service Data Analytics?
Self-Service Data Analytics can be challenging. It involves managing data consistency, privacy, user skill gaps, regulatory compliance, and integration with existing systems.
3. What are some future trends in Self-Service Data Analytics?
Self-Service Data Analytics has drastically evolved and is set to continue growing. In the future, we can expect a surge in the use of artificial intelligence and machine learning, increased focus on predictive and mobile analytics, and more emphasis on effective data governance.
Let’s Talk About What MarkovML
Can Do for Your Business
Boost your Data to AI journey with MarkovML today!