Mastering Data Profiling: Techniques for Enhanced Data Analysis
Data profiling, a critical component in data analysis, involves scrutinizing and understanding data sets to ensure their quality and appropriateness for specific tasks. This process is integral in today's data-driven world, where accurate data analysis underpins decision-making across various industries.
Data profiling facilitates the identification of patterns, anomalies, and inconsistencies, enabling organizations to make informed decisions. Whether in machine learning, artificial intelligence, or traditional data analytics, the significance of data profiling cannot be overstated.
The ESG 2023 State of Data Intelligence Report shows that organizations with fully implemented data intelligence strategies are more likely to recognize the impact of data profiling and quality scoring. This highlights the growing reliance on data-driven decision-making in companies, with data profiling being a pivotal element.
In this blog post, you will learn about the techniques for data profiling and understanding data.
Data Profiling Techniques
Data profiling techniques are crucial for extracting meaningful insights from data and facilitating informed decision-making across various business functions.

It helps organizations leverage data effectively, driving strategic advantages in an increasingly data-centric world.
Here are the five topmost data profiling techniques:
1. Descriptive Statistics
It involves summarizing data using measures like mean, median, mode, and standard deviation. For instance, calculating the mean and standard deviation of sales data can help identify trends and understand the typical performance levels.
2. Data Visualization
This approach makes complex data more accessible through visual representation, such as charts and graphs. An example is plotting a histogram to visualize the age distribution of customers, which can reveal demographic trends.
3. Data Structure Analysis
It involves examining how different elements of data are related. For example, mapping relationships between product categories and sales can uncover which products are often bought together.
4. Data Quality Metrics
It assesses the quality of data by evaluating aspects like accuracy, completeness, and consistency. An example is checking for missing values in customer records to evaluate data completeness.
5. Pattern Recognition
This technique involves identifying and analyzing patterns within data. Detecting irregular purchasing behavior using pattern recognition in transaction data, for instance, can help identify fraudulent activities or unique buying trends.
Data Profiling Steps
A structured approach to data profiling is essential for accuracy and efficiency. It ensures that data is thoroughly examined and that the insights derived are reliable and actionable.
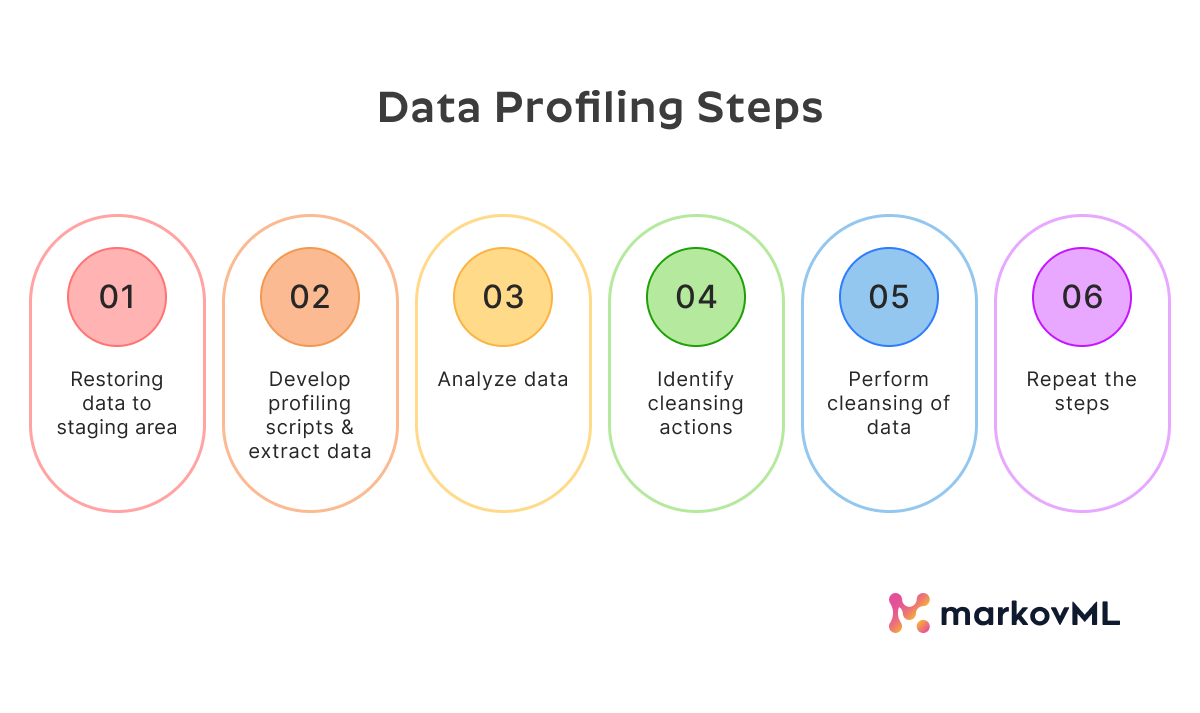
This systematic method reduces errors and biases, leading to more informed decision-making.
Systematic Steps in Data Profiling
- Data Collection: Gathering relevant data from various sources.
- Data Cleaning: Identifying and correcting errors or inconsistencies in the data.
- Data Structure Analysis: Understanding the relationships and patterns within the data structure.
- Data Quality Assessment: Evaluating the quality of data in terms of accuracy, completeness, and consistency.
- Data Visualization: Representing data visually to identify trends and insights more effectively.
Data Profiling Challenges
Navigating the complexities of data profiling requires addressing key data profiling challenges that can hinder the process. This includes:
1. Data Inconsistencies
Variations in data formatting across different systems can lead to errors. For example, one system might record dates as DD/MM/YYYY, while another uses MM/DD/YYYY.
2. Data Privacy Concerns
Ensuring compliance with data protection regulations (like GDPR) is challenging, especially when profiling sensitive personal data.
3. Data Volume
The sheer amount of data can be overwhelming, making it difficult to process and analyze it efficiently.
4. Missing Data
Incomplete datasets can skew analysis results. For instance, missing income data in customer records can lead to inaccurate customer segmentation.
Data Profiling Best Practices
To overcome the above-mentioned data profiling challenges, businesses can adopt certain best practices to ensure a more efficient and accurate data profiling process:
1. Data Standardization
Implementing uniform data formats across systems. For example, standardizing date formats to YYYY-MM-DD.
2. Regular Data Audits
Periodically reviewing data for errors or inconsistencies to maintain quality.
3. Data Quality Framework
Establishing clear guidelines for data quality, such as accuracy and completeness metrics.
4. Data Governance
Developing policies and procedures for data management and usage to ensure data integrity and compliance.
The Role of Data Profiling
Data Profiling plays a huge role in business decision making which have a significant impact in achieving good quality results and profit.
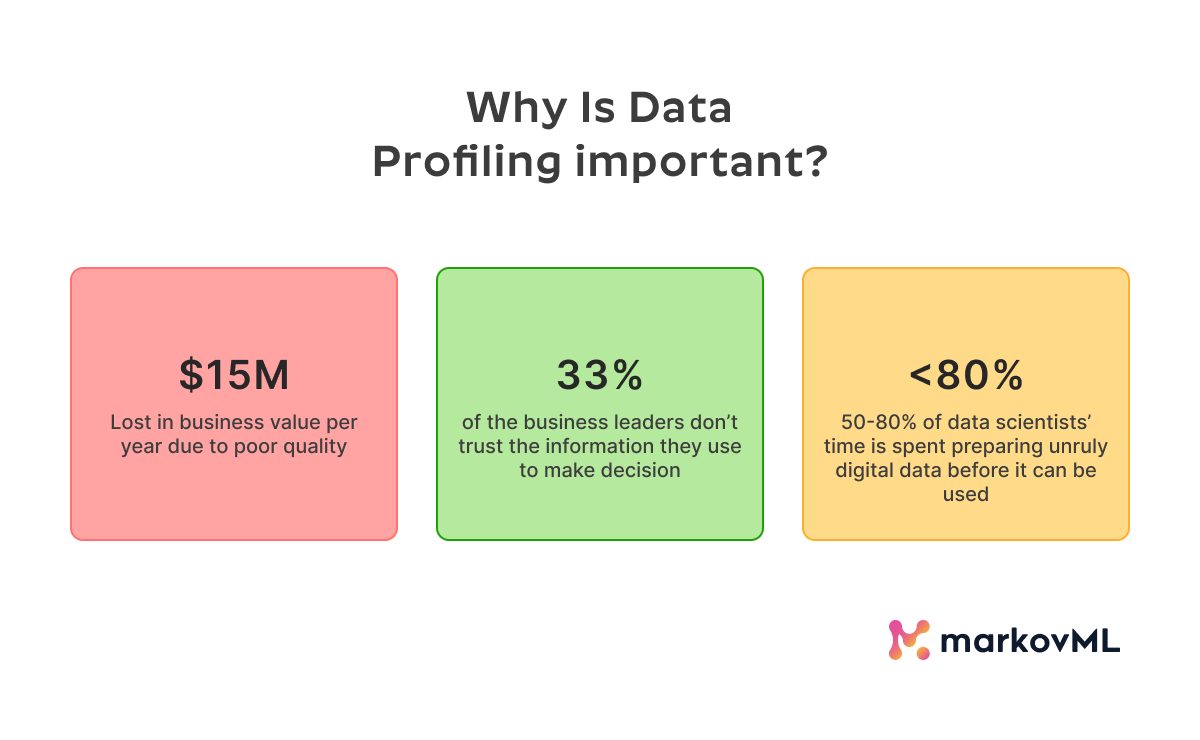
The Role of Data Profiling in Decision-Making
Data profiling significantly enhances decision-making by providing accurate, high-quality data.
For instance, in retail, profiling customer data helps in tailoring marketing strategies to specific demographics, thereby improving campaign effectiveness and ROI.
The Role of Data Profiling in Machine Learning and AI
In Machine Learning and AI, data profiling is pivotal for training accurate models.
For example, in predictive maintenance, profiling machine performance data ensures the model is trained on accurate, relevant data, leading to more precise predictions and reduced downtime. This role underscores the importance of clean, well-profiled data in AI-driven solutions.
Conclusion
Data profiling stands as a cornerstone in the realm of data analysis, vital for informed decision-making and optimizing strategies in various industries. It serves as the foundation for training robust models.
Additionally, data profiling tools like Markov offer a practical, no-code platform for data analysis, app building, and AI workflow management, simplifying the data profiling process and empowering teams with efficient, data-centric solutions.
This integration of sophisticated techniques and data profiling tools like Markov paves the way for businesses to harness the full potential of their data, driving innovation and success in the data-driven era.
Want to know more about what Markov has to offer? Book a demo today!
FAQs
1. What is the primary purpose of data profiling?
Data profiling aims to understand and improve the quality of data. It involves analyzing data sets to identify patterns, inconsistencies, and anomalies, thereby ensuring the data's suitability for specific tasks or decision-making processes.
2. How does data profiling aid in machine learning?
In machine learning, data profiling is crucial for preparing accurate and reliable datasets. It helps in identifying and rectifying data quality issues, ensuring that machine learning models are trained on clean, relevant data for better performance and accuracy.
3. Can data profiling help in regulatory compliance?
Yes, data profiling plays a significant role in regulatory compliance. Ensuring data quality and consistency helps organizations adhere to data protection and privacy regulations, like GDPR, by identifying and addressing potential compliance issues in their data sets.
Let’s Talk About What MarkovML
Can Do for Your Business
Boost your Data to AI journey with MarkovML today!