Unlocking Innovation: Collaborative Machine Learning with AI
Machine learning processes involve vast volumes of data that organizations use to derive critical insights from. These insights help shape their understanding of customer behavior and product viability and help organizations innovate further.
However, with these vast amounts of data comes the need for various teams to collaborate to ensure a project's success.
This is where AI can play a crucial role, streamlining communication among teams and their respective members while ensuring everyone operates on the latest available data. AI opens the door to generative AI apps, smart data management, and much more.
The benefits of this collaboration between AI and ML are immense, and this guide will explore how AutoML tools can help you maximize productivity and innovation.
Understanding Collaboration in ML With AI
The benefits of AI are being felt across industries today, and it's playing a crucial role in ensuring the success of collaborative machine learning operations. Some of the advantages of using AI in collaborative machine-learning tasks include the following:
- Improved Data Quality: Streamline data quality checks by checking for missing information, values, and any possible biases.
- Data Versioning: This ensures individuals always operate with the latest available information for the best possible results.
- Selecting ML Models: It can help select the best machine learning models based on the data available and the task at hand, thereby ensuring accurate and reliable results.
- Streamlined Communication: It enables the provision of a centralized platform to help streamline collaboration and ensure seamless communication across teams.
As you can see, effectively collaborating in ML with AI can streamline a wide range of operations. It offers means and ways of making complex tasks easier to work on by offering seamless collaboration across large teams.
Additionally, it also enables teams to work with the latest information at all times, thereby ensuring the most accurate results possible.
AI-Enhanced Collaboration Platforms and Tools
AI and ML collaboration tools offer businesses the platform they need to enable extensive collaboration among every stakeholder in ML processes across the organization. Each member can add changes that reflect in real-time, allowing for efficient revisions and no scope for duplication.
Intelligent Collaborative Notebooks
Notebooks are one of the key features that enable real-time collaboration between ML teams. With a clear set of goals, these teams can utilize notebooks to collaborate and align various aspects of a project seamlessly.
For example, you can add a Python file, a text file, and a Markdown file, among others, which is available to every member.
With access to all relevant information, AutoML can automatically preprocess datasets, select the right machine-learning models, and deploy them when needed. These features significantly improve performance while increasing operational efficiency.
AI-Enhanced ML Model Development Platforms
AutoML tools offer a wide array of machine-learning models to choose from. What's more, the tools offer the best-suited models for your dataset using elements and techniques like performance metrics and cross-validation. This helps maximize efficiency, as teams no longer have to manually try out various models to find the most suitable one.
These tools can then also provide a seamless means of deploying the algorithm once it's been tweaked and fine-tuned. As a result, developers and data scientists can maximize productivity as AutoML takes care of a range of labor-intensive tasks.
A key example of the use of AutoML is in medical diagnosis management. A combination of AI and ML can gather data based on various parameters such as a forecast of a disease's progression, treatment planning, medical support, and so on, preventing the need for human intervention in performing such manual tasks.
Collaboration Challenges in ML With AI
While AI in ML offers a range of benefits, it also poses certain challenges, some of which are mentioned below.
- Requires High-Quality Data: Machine learning algorithms need to be built on a foundation of high-quality data, and if this is missing, the algorithm may produce suboptimal results. As a result, the data you input must be detailed, non-biased, and as comprehensive as possible.
- Needs High Computational Performance: Successful use of AutoML requires high-performance computational resources, especially when running complex model selection algorithms or optimizing hyperparameters. This can often prove to be challenging for smaller teams with a limited budget.
- Automation Over Customizability: While AutoML automates several processes, this can come at the cost of the ability to customize algorithms, which several experts often prefer. As a result, they can make it challenging to incorporate some advanced knowledge or unconventional techniques.
- Makes Processes Seem Simpler Than They Are: Last but not least, AutoML can also lead to a false perception of the process's simplicity, leading many to believe that it's okay to have no or limited knowledge of how machine learning algorithms work.
Best Practices for Collaborative ML With AI
Utilizing AutoML requires certain best practices for AI-driven collaboration to ensure you utilize the technology's potential to the fullest, some of which include:
- Define Clear Goals: The first thing to do is to define clear goals and roles for every member of the team. This also involves a clear direction on how to measure the success of your operations. This clarity ensures that everyone's contribution meets what's expected of them, with no room for confusion.
- Streamline Workflows: Adopt a standard workflow to streamline each process, from data collection to pre-production, modeling, and deployment, as this ensures a structured approach to the project.
- Communicate at All Times: One of the key highlights of AutoML is that it allows you to communicate using Snippets. This must be utilized to ensure timely communication and feedback.
- Leverage the Best Tools: This can help you automate your machine-learning processes and streamline communication. These tools enable communication in real-time and utilize version control to ensure there's no duplication of work, thereby ensuring all the resources diverted to the project are utilized efficiently.
A collaboration between AI and ML benefits companies in terms of five key principles:
- Reimagining business processes
- Embracing employee involvement
- Actively directing AI strategy
- Collecting data responsibly, and
- Redesigning the process to incorporate the use of AI.
The manufacturing sector is a key example of where the use of AI and ML offers significant benefits. From automating and optimizing the manufacturing process to managing supply chains and on-demand production, a manufacturing unit can transform its operations.
What's more, the use of data collected via AI has helped businesses redesign their processes to achieve greater efficiency and facilitate a better working relationship between technology and human labor. The same principles also apply to sectors like banking, retail, and so on.
Case Studies in Collaborative ML With AI
One of the key case studies that shows the potential of an AI-driven collaboration is that of OneSoil. The key challenge was to offer farmers the critical information they need to make predictions about crop diversity, weather patterns, and more.
Without the resources and technology to predict these events, farmers are typically left at the mercy of natural or other factors.
The solution provided via the OneSoil app uses machine learning and computer vision to provide farmers with critical information that allows them to predict outcomes and make informed decisions. The data is collected from the European Commission's satellite program, the Copernicus, and from sensors on farms.
Collaboration between multiple stakeholders, from farmers to machine operators and managers, allowed farmers to predict factors like their farms' productivity, the emergence of potential pests and diseases, and much more. This, in turn, allows them to manage their fieldwork effectively.
Future Trends in Collaborative ML With AI
Collaborative ML with AI is set to see tremendous growth over the next few years. In fact, according to Proficient Market Insights, the market for machine learning platforms is set to reach $31,360 million by 2028. With this in mind, and with the predicted growth of AI as well, there are several key trends in collaborative ML with AI to look out for. Some of these include:
- High-Quality Personalized Learning: An increase in the quality of personalized learning, with machine learning algorithms being able to optimize the learning process, provide personalized tutoring, and a whole lot more.
- Analyzing Large Data Sets: The ability to analyze vast amounts of data in medical diagnostics, treatment planning, and drugs.
- Improved Customer Service: An improvement in customer service, with the use of advanced virtual assistants and other tools to help streamline operations and improve customer satisfaction.
- AI and ML in Autonomous Vehicles: The rise of autonomous vehicles, with AI and ML being able to process vast amounts of data to improve road safety and revolutionize logistics and delivery systems.
The infographic below should give you an idea of the scope of growth AI presents.
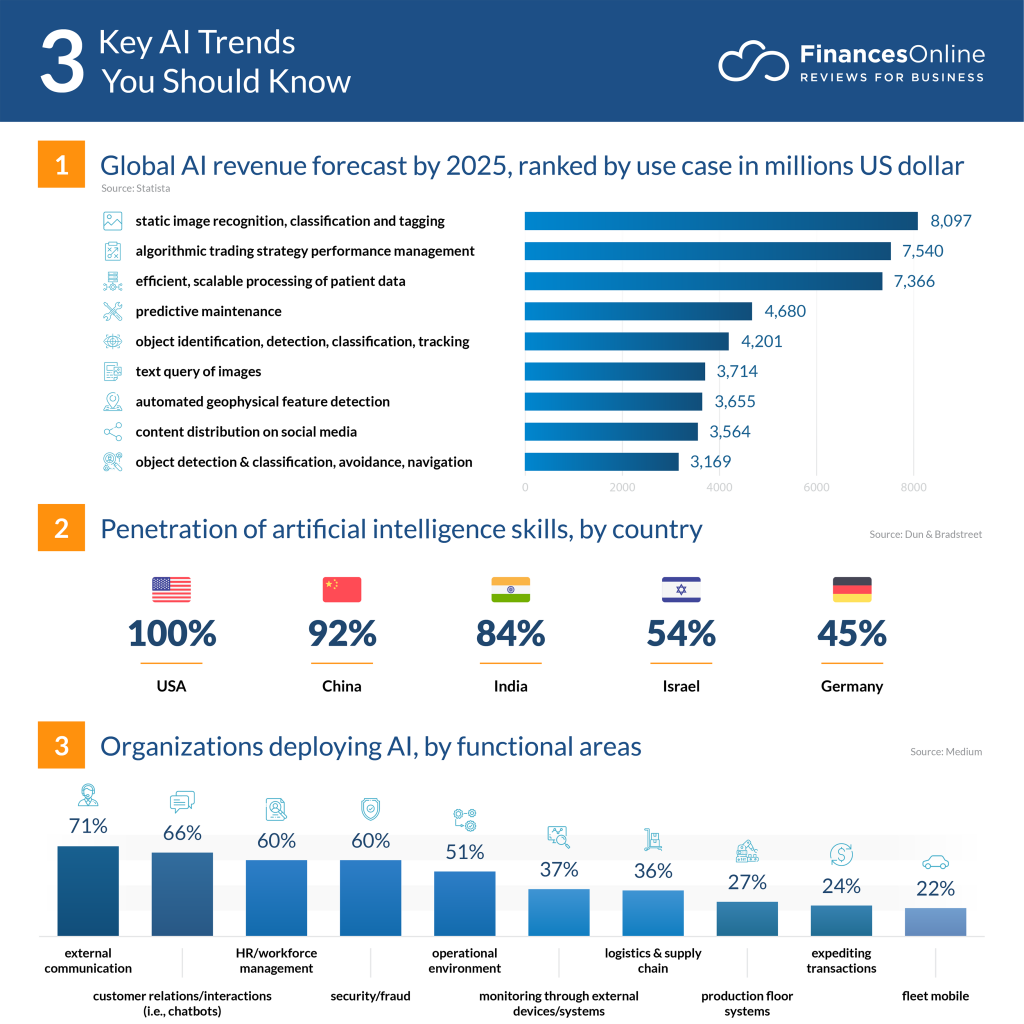
In Conclusion
The combination of AI and collaborative machine learning offers endless possibilities across various sectors such as education, healthcare, technology, logistics, and more. AutoML plays a major role in democratizing machine learning and making it available to a larger number of individuals and organizations, thereby incentivizing innovation.
Its ability to automate processes while allowing for real-time communication and feedback goes a long way in improving operational efficiency and communication and enabling a more strategic allocation of experienced resources.
If you're looking to leverage the power of collaborative ML with AI but are held back by limited accessibility, MarkovML can help you achieve your goals with purpose-built tools. Reach out to find out how we can help you streamline your organization's operations and maximize efficiency.
Don't forget to check out MarkovML's blog for more detailed guides and insights into the potential of collaborative machine learning with AI.
Let’s Talk About What MarkovML
Can Do for Your Business
Boost your Data to AI journey with MarkovML today!