13 Data Quality Best Practices You Should Adopt In 2024
In the digital age, data reigns supreme, shaping decisions, driving innovations, and underpinning the success of organizations across industries. Yet, the reliability of these data treasures hinges on a critical factor—data quality.
Did you know that data quality issues cost the US economy an estimated $3.1 trillion per year?
According to Harvard Business Review, 94% of companies say data is critical to growing their business, yet only 3% of companies' data meets basic data quality standards. These statistics lead to an important realization: most companies struggle to maintain high data quality, highlighting the urgent need for a data quality checklist.
The Landing AI co-founder and CEO, Andrew Ng says, “The key is better data, not bigger data”, and at Markov, we cannot agree more.
Hence, in this blog, we talk about 13 data quality best practices businesses should adopt in 2024. We also delve into the core principles of data quality and its profound impact on the bottom line. From the fundamentals of data quality to strategies for ensuring it, we uncover the key to unlocking data's full potential.
To top it, we examine real-world success stories, highlighting how businesses like Amazon have thrived by making high data quality a cornerstone of their operations. Let’s dive in!
Table Of Contents
- What Is Data Quality And Why Does It Matter
- How To Ensure Data Quality
- Data Quality vs. Data Integrity
- What Are The Elements Of Data Quality
- How To Define Data Quality Metrics And KPIs
- 13 Data Quality Best Practices
- Amazon's Data-Driven Success
- Conclusion
What Is Data Quality And Why Does It Matter
Data quality refers to the accuracy, completeness, consistency, reliability, relevance, and timeliness of data within an organization.
It signifies the trustworthiness of data for making informed decisions and conducting business operations. Data quality is vital because it directly influences the reliability of insights and decisions derived from data.
Poor data quality can lead to costly errors, misinformed strategies, and damaged reputations. Conversely, high data quality enhances confidence in data-driven decisions, boosts operational efficiency, and supports compliance with regulatory requirements.
In today's data-driven landscape, data quality is an indispensable asset underpinning organizations' success and competitiveness across industries.
How To Ensure Data Quality
Ensuring good data quality is a multifaceted endeavor crucial for maintaining the integrity of your organization's data assets. Here are key strategies to achieve this:
1. Data Profiling: Begin by thoroughly analyzing your data to identify inconsistencies, errors, and anomalies. Data profiling tools can help automate this process, providing valuable insights into data quality issues.
2. Data Validation: Implement validation rules and constraints to ensure data accuracy at the point of entry. This can include format checks, range validations, and referential integrity constraints.
3. Data Cleansing: Regularly cleanse your data to remove duplicate records, correct inaccuracies, and standardize formats. Automated data cleansing tools can streamline this process.
4. Data Standardization: Establish clear data standards and data dictionaries to ensure uniformity in data representation. This helps in maintaining consistency across different data sources.
5. Data Quality Tools: Leverage data quality software and tools that provide real-time monitoring, alerting, and reporting on data quality issues. These tools can help you proactively address problems as they arise.
6. Data Quality Metrics: Define and track key performance indicators (KPIs) related to data quality. This allows you to measure progress and identify areas needing improvement.
7. Data Governance: Develop a robust data governance framework with clear roles and responsibilities for data stewardship. This ensures accountability for data quality throughout the organization.
8. Data Auditing: Conduct regular data audits to assess the quality of your data against established standards. Audits should encompass data completeness, accuracy, reliability, and relevance.
9. Data Training: Provide training to staff on the importance of data quality and best practices for data entry, maintenance, and validation.
10. Data Quality Culture: Foster a culture within your organization that values and prioritizes data quality. Make data quality a shared responsibility across departments.
11. Data Quality Policies: Create and enforce data quality policies and procedures, including data retention and archiving policies.
12. Data Quality Assurance Team: Consider forming a dedicated data quality assurance team responsible for monitoring, assessing, and continuously improving data quality.
By implementing these strategies, and the data quality best practices mentioned below, organizations can ensure that their data remains a trusted and valuable asset, supporting informed decision-making and enhancing overall operational efficiency.
Data Quality vs. Data Integrity
Data quality and data integrity are both critical aspects of effective data management, each with its unique focus and significance in ensuring data reliability and usability.
While data quality pertains to the overall fitness and usefulness of data, data integrity emphasizes the consistent and accurate state of data over time, safeguarding it from corruption or unauthorized changes.
To create a table with the provided columns and data in Webflow, you can use HTML and CSS. Here's the code to create the table:
You can add this HTML code to your Webflow project by using an Embed element. Simply drag and drop an Embed element into your Webflow page, and then paste the code inside it. You can further style the table using Webflow's built-in styling options or by adding custom CSS if needed.
AspectData QualityData IntegrityDefinitionThe overall fitness of data for its intended use.The consistency and accuracy of data over time.FocusAccuracy, completeness, reliability, relevance, timeliness, and validity.Consistency, reliability, and accuracy.ScopeBroader scope, encompassing various data attributes.Primarily concerned with data accuracy and consistency.ImpactInfluences decision-making, operational efficiency, and trustworthiness.Ensures data remains reliable and consistent over time.MonitoringOngoing monitoring and maintenance are essential.Focuses on preventing data corruption and unauthorized changes.ExamplesData quality issues include missing values, duplicate records, and incorrect data.Data integrity issues include data corruption, unauthorized access, and tampering.
What Are The Elements Of Data Quality
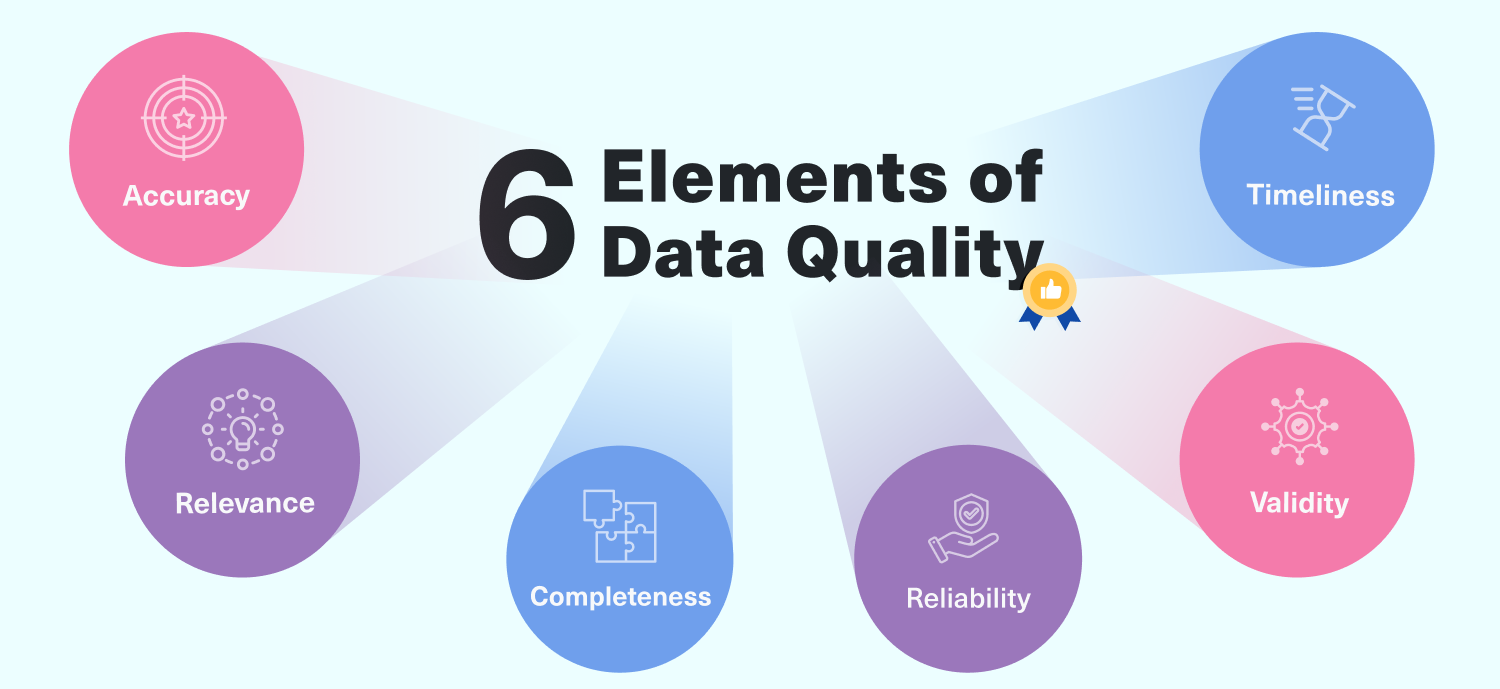
1. Accuracy: Accuracy refers to the degree to which data correctly represents the real-world objects or events it is meant to describe. High accuracy ensures that data reflects the truth and minimizes errors in analysis and decision-making.
2. Completeness: Completeness measures whether all required data elements are present in a dataset. Data sets lacking crucial information can lead to incomplete analyses and hinder the ability to make informed decisions.
3. Reliability: Reliability assesses the consistency and stability of data over time. Reliable data can be trusted to remain unchanged and consistent, allowing for accurate historical analysis and decision-making.
4. Relevance: Relevance gauges how well data aligns with the specific purpose for which it is intended. Relevant data is essential for making informed decisions, as irrelevant information can lead to confusion and incorrect conclusions.
5. Timeliness: Timeliness reflects the currency of data—how up-to-date it is. Timely data ensures that decisions are based on current information, particularly crucial in fast-paced environments.
6. Validity: Validity measures whether data conforms to defined rules and constraints. Valid data adheres to specified formats, ranges, and standards, enhancing its usefulness and reliability in various applications.
How To Define Data Quality Metrics and KPIs
Measuring data quality involves assessing the fitness of data, and ensuring it meets predefined standards and criteria.
Key steps in measuring data quality and data quality control include defining specific data quality metrics and key performance indicators (KPIs) tailored to your organization's needs. These metrics might assess accuracy, completeness, reliability, relevance, timeliness, and validity.
Automated data quality assessment tools like MarkovML can automate the profiling process, providing insights into data quality issues. User feedback, regular audits, and data quality scores can also contribute to the measurement.
Check Your Data Quality Score With MarkovML
The goal is to quantify the state of data quality, identify areas for improvement, and maintain a high standard of data integrity for informed decision-making.
13 Data Quality Best Practices to Adopt in 2024
In the data-driven landscape of 2024, ensuring data quality is paramount. Explore these 13 best practices to maintain accurate, reliable, and valuable data, enabling organizations to make informed decisions and stay competitive in an increasingly data-centric world.
1. Establish How Improved Data Quality Impacts Business Decisions
Understanding the direct link between data quality and business outcomes is crucial. It involves quantifying how data improvements lead to better decision-making, reduced errors, and increased profitability. This helps garner support and resources for data quality initiatives.
2. Define "Good Enough" Data Quality Standards
Establishing clear criteria for acceptable data quality levels is vital. Organizations must define what constitutes "good enough" data quality, aligning expectations and resources with realistic goals to avoid overinvestment in perfection.
3. Standardize Data Quality Across the Organization
Ensure that data quality practices are consistent throughout the organization, spanning all departments and teams. This consistency promotes uniformity and reliability in data management.
4. Utilize Data Profiling Regularly
Regular data profiling involves analyzing data to identify quality issues proactively. By regularly assessing data, organizations can pinpoint and address problems early, preventing them from compounding over time.
5. Implement Data Quality Dashboards
Data quality dashboards provide real-time monitoring of critical data assets, such as master data. These dashboards enable organizations to detect and address quality issues promptly, ensuring data remains reliable and trustworthy.
6. Shift from Truth-Based to Trust-Based Semantic Models
Embracing trust-based semantic models means prioritizing data that aligns with real-world scenarios. By fostering trust in your data, organizations can confidently rely on it for decision-making.
7. Include Data Quality Best Practices in Governance Board Meetings
Make data quality a recurring agenda item in governance board meetings to emphasize its importance. This ensures that data quality remains a top priority in organizational decision-making.
8. Assign Data Quality Responsibilities to Data Stewards
Clearly define roles and responsibilities for managing data quality within the organization. Data stewards play a vital role in ensuring that data quality standards are met and maintained.
9. Form a Special Interest Group for Data Quality
Foster collaboration between business units and IT by establishing a special interest group dedicated to data quality. This collaboration helps drive data quality improvements across the organization.
10. Incorporate Data Quality Reviews in Release Management
Integrate data quality checks into the release management process. This ensures that data quality is upheld as a crucial component of new data releases.
11. Regularly Communicate Data Quality Benefits
Continuously educate stakeholders about the positive impacts of data quality efforts. This helps maintain enthusiasm and support for ongoing data quality initiatives.
12. Engage with External/Industry Peer Groups
Learn from industry peers and stay updated on best practices through engagement with external and industry-related forums. These interactions provide valuable insights and benchmarking opportunities.
13. Iterate and Continuously Improve Data Quality Processes
Data quality is an ongoing process. Regularly assess and refine data quality procedures to adapt to changing data environments and ensure sustained high quality data.
Amazon's Data-Driven Success Story
Amazon, one of the world's largest e-commerce and technology companies, has achieved remarkable business success through its relentless focus on improving data quality.
Their Challenge:
In the early 2000s, as Amazon expanded its product offerings and customer base, the company faced the challenge of managing vast amounts of data efficiently. With millions of products, customer reviews, and third-party sellers on its platform, maintaining accurate and reliable data became paramount.
The Solution:
Amazon invested heavily in data quality initiatives, implementing a range of practices like:
- Data Cleansing: Amazon developed sophisticated data cleansing algorithms to identify and rectify errors, inconsistencies, and duplicate product listings. This not only improved the customer experience but also reduced operational inefficiencies.
- Recommendation Systems: The company harnesses high quality data to enhance its recommendation algorithms. By analyzing customer behavior and purchase history, Amazon could provide personalized product recommendations, increasing sales and customer satisfaction.
- Supply Chain Optimization: Accurate data about inventory levels, order status, and shipping times allowed Amazon to optimize its supply chain operations. This resulted in faster deliveries, reduced costs, and improved customer trust.
The Outcome:
The commitment to data quality had a transformative impact on Amazon's business in the following ways.
- Increased Sales: Accurate product data and improved recommendations led to increased sales and customer loyalty, contributing significantly to Amazon's revenue growth.
- Operational Efficiency: Better data quality streamlined internal processes, reducing errors, and saving costs in areas like inventory management and order fulfillment.
- Market Dominance: Amazon's ability to leverage high quality data gave it a competitive edge, allowing the company to expand into new markets and industries beyond e-commerce, such as cloud computing with Amazon Web Services (AWS).
Amazon's success story illustrates how prioritizing data quality and leveraging data-driven insights can lead to remarkable business achievements, from revenue growth and operational efficiency to market dominance and diversification.
Conclusion
Good data quality is not a static concept but a dynamic process that demands vigilance, dedication, and strategic planning. Understanding how to maintain data quality and embracing the 13 data quality best practices outlined in this blog can empower organizations to harness the full potential of their data in 2024 and beyond.
From aligning data quality with business decisions to fostering a culture of trust in data, these practices offer a comprehensive roadmap for success. By prioritizing data quality, organizations can make more informed decisions, streamline operations, and maintain a competitive edge in our increasingly data-driven world.
Let’s Talk About What MarkovML
Can Do for Your Business
Boost your Data to AI journey with MarkovML today!