Mastering Data Quality: Strategies and Tools for Business Success in 2024
The Experian 2021 Global Data Management Research report highlights the tangible consequences of poor data quality:
- Wasted resources and escalated costs (40%)
- Compromised analytics reliability (36%)
- Eroded customer trust and reputation (32%)
- A negative customer experience (32%)
- A slowdown in digital transformation (31%)
Addressing these challenges not only averts these pitfalls but also fosters reliable analytics, streamlined operations, and cost reduction.
Recognizing this, Gartner states that almost 70% of organizations already rigorously track data quality metrics. This trend underscores a practical, results-oriented approach to data management. And that’s exactly what we’re going to talk about in this blog.
Understanding Data Quality
Data quality, fundamentally, refers to the accuracy, consistency, completeness, reliability, and timeliness of data. Its significance is paramount in decision-making processes, where high-quality data underpins sound business strategies.
.webp)
For instance, in the healthcare industry, impeccable data quality ensures accurate patient records, directly influencing treatment efficacy and patient safety. In contrast, in the retail sector, superior data quality translates into precise customer insights, driving tailored marketing strategies and enhancing customer satisfaction.
How Data Affects Various Industries
- Healthcare: Accurate patient data is crucial for effective treatments and patient safety.
- Retail: High-quality data drives personalized marketing and improves customer satisfaction.
- Finance: Precise data is essential for accurate risk assessment and regulatory compliance.
- Manufacturing: Reliable data is key to accurate production planning and inventory management.
- Education: Good data quality supports tailored educational programs and student success tracking.
- Technology: In tech industries, data quality impacts innovation speed and product reliability.
- Real Estate: Quality data aids in accurate property valuation and market trend analysis.
- Transportation: Dependable data ensures efficient route planning and logistic management.
- Government: High data quality is vital for informed policy-making and public service delivery.
- Tourism and Hospitality: Quality data helps in tailoring guest experiences and service improvements.
The Data Quality Lifecycle
The data quality lifecycle encompasses several critical stages, each demanding stringent quality maintenance to ensure overall data integrity and utility.
Stage 1: Data Collection
The initial stage involves gathering data, where accuracy is paramount for foundational integrity.
Poor collection practices can lead to initial data quality challenges, necessitating immediate improvement measures. For example, in retail, inaccurately captured customer data can mislead future marketing efforts, demonstrating the need for effective data quality assessment tools right from the start.
Stage 2: Data Storage
Post-collection data is stored for future use. Maintaining data quality here is crucial to prevent corruption or loss.
In finance, secure and accurate data storage is a necessity for compliance. Over time, storage systems must adapt to evolving data quality technology trends to prevent degradation.
Stage 3: Data Processing
At this stage, data is transformed into a usable format. Quality control during processing is vital to avoid errors that can multiply.
In healthcare, accurate processing of patient data is essential, highlighting the importance of robust data quality software and monitoring to track and manage these transformations.
Stage 4: Data Analysis
The final stage is where data is analyzed for decision-making. Maintaining quality here ensures reliable insights.
For example, in marketing, high-quality data analysis is necessary for effective campaigns. However, data quality can degrade due to multiple processing steps, emphasizing the need for continuous data quality management and metrics to ensure ongoing reliability.
Common Data Quality Issues
Common data quality issues often hinder effective decision-making and operational efficiency in businesses.
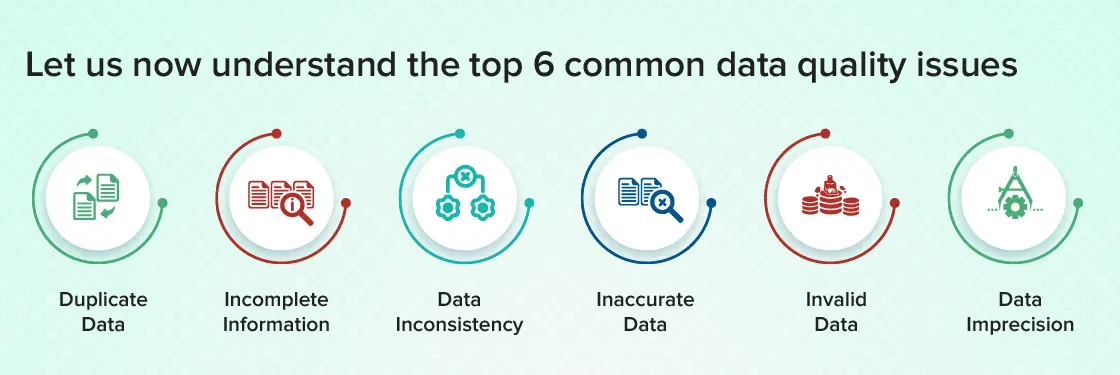
Addressing these is crucial for data quality improvement and management.
1. Duplicates
Duplication of data entries can lead to inefficiency and skewed analysis. For instance, duplicate customer records in a CRM system can result in redundant marketing efforts, wasting resources. Implementing data quality software that identifies and merges duplicates is a practical solution.
2. Inaccuracies
Incorrect data entries lead to misguided decisions. A financial institution relying on inaccurate credit scores could extend or deny loans inappropriately. Regular data quality assessment and monitoring can help in identifying and correcting inaccuracies.
3. Missing Values
The absence of critical data can render datasets incomplete, affecting the analysis. In healthcare, missing patient history details can lead to inadequate care plans. Utilizing data quality tools to identify and address missing values is essential.
4. Inconsistencies
Discrepancy in data across different systems can cause confusion. For example, inconsistent pricing information in retail across various platforms can lead to customer distrust. Implementing data quality management protocols and using metrics to ensure consistency across platforms can alleviate this issue.
Data Quality Assessment and Measurement
Assessing data quality requires a structured approach using defined metrics and standards.
.webp)
Here’s how you can go about it:
Step 1: Define Data Quality Criteria
Establish clear criteria for what constitutes quality data in your context.
Example: A retail business might set accuracy, completeness, and timeliness as key quality parameters for customer data to ensure effective targeting in marketing.
Step 2: Select Appropriate Measurement Tools
Choose tools that align with your data type and industry-specific needs.
Example: In finance, tools that specialize in detecting inaccuracies in transactional data are crucial.
Step 3: Implement Relevant Metrics for Assessment
Apply specific metrics to evaluate data quality regularly.
Example: Error rates and completeness percentages can be used in healthcare to assess patient data quality, improving care and operational efficiency.
Step 4: Continuous Monitoring and Improvement
Integrate continuous monitoring into the data quality lifecycle for ongoing management and improvement.
Example: Regular monitoring in healthcare ensures patient data remains accurate and up-to-date, directly impacting patient treatment and care.
Technology Solutions for Ensuring Data Quality
Leveraging the right technologies and tools is crucial for ensuring and improving data quality. The following tools not only address data quality challenges but also enhance the overall data quality lifecycle and management.
A. Data Quality Software
Comprehensive software solutions automate many aspects of data quality control, such as detecting inconsistencies and duplicates. They are essential for businesses looking to streamline their data quality improvement processes.
B. Data Profiling Tools
These tools analyze datasets to provide insights into data quality, structure, and patterns, helping in identifying areas that require attention.
C. Data Validation Tools
Essential for ensuring data integrity, these tools check data against specific rules or standards, which are critical for maintaining accuracy, especially in sectors like finance and healthcare.
D. Data Quality Dashboards
Dashboards provide a visual representation of data quality metrics, making it easier to monitor and manage data quality in real time.
E. Data Enrichment Tools
By augmenting existing data with additional information, these tools enhance the completeness and relevance of the data, particularly useful in marketing and customer relationship management.
F. Data Governance Platforms
These platforms provide a framework for data management, ensuring compliance with data standards and policies across the organization.
G. Machine Learning for Data Quality
Innovative tools like Markov use machine learning algorithms to predict and rectify data quality issues. They can adapt to changing data patterns and are particularly effective in environments with large, complex datasets.
Conclusion
It all narrows down to two things:
- Defining the right strategy specific to your business and industry needs.
- Finding the right tool that helps you apply these strategies.
When selecting the right tool, consider factors like the specific data quality challenges faced, the volume and complexity of your data, and the industry you operate in. For instance, Markov’s Label Quality Score enables you to quantify the label quality of your data, so that you can work towards bettering it.
This feature from Markov is particularly beneficial for organizations looking to streamline their data handling processes, ensuring data quality throughout its lifecycle.
FAQs
1. What are the most common data quality issues and their impact on businesses?
Issues like duplicates, inaccuracies, missing values, and inconsistencies are common. For instance, duplicates in a CRM can lead to redundant marketing efforts, escalating costs, and resource wastage.
2. How does data quality affect different industries?
Data quality impacts industries uniquely. In healthcare, poor data quality can compromise patient safety, while in finance, it may lead to incorrect risk assessments, affecting compliance and financial stability.
3. What role do modern technologies like Markov play in data quality management?
Technologies like Markov, with its AI-driven data analysis and no-code GenAI app builder, streamline data quality improvement, offering robust solutions for managing complex datasets and enhancing overall data governance.
Let’s Talk About What MarkovML
Can Do for Your Business
Boost your Data to AI journey with MarkovML today!